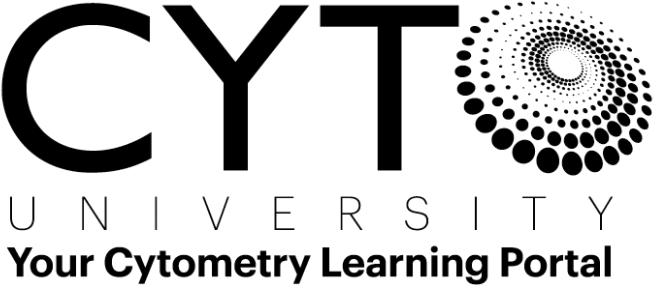
Don’t Leave Home Without a Map: Powerful Dimensionality Reduction Methods for Cytometry Data Visualization
-
Register
- Visitor - $50
- Bronze - Free!
- Silver - Free!
- Gold - Free!
- Platinum - Free!
- Community Administrator - Free!
- ISAC Staff - Free!
- Bronze Lab Membership - Free!
- Silver Lab Membership - Free!
- Platinum Lab Membership - Free!
About the Presenter
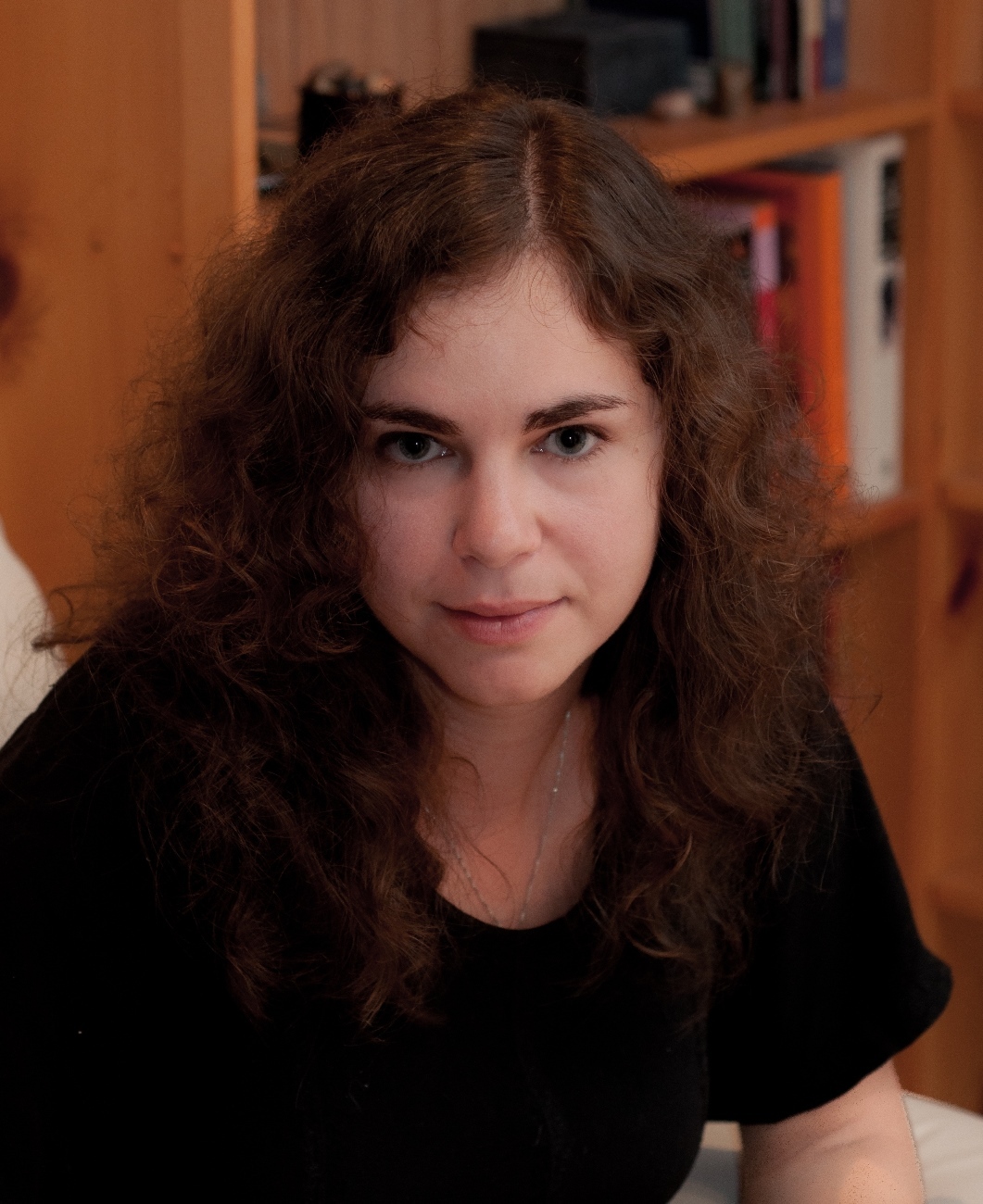
Anna Belkina, MD, PhD
Assistant Professor of Pathology and Laboratory Medicine
Director, Flow Cytometry Core Facility
Boston University School of Medicine
Anna C. Belkina is an assistant professor of pathology and laboratory medicine and the director of the Flow Cytometry Core Facility at Boston University School of Medicine. She received her MD degree from Russian State Medical University in Moscow and her PhD degree from Boston University School of Medicine investigating the epigenetic regulation of inflammatory responses driven by bromodomain proteins. Anna’s research is focused on the intersection of immunology and computational biology and her research efforts include investigating the immune landscape of chronic inflammatory diseases and developing computational techniques to assess high-parameter single cell cytometry data. She has designed the opt-SNE algorithm that is now widely used for the visualization of multidimensional cytometry datasets.
Anna is an active member of ISAC and has been named a 2015–2019 ISAC SRL Emerging Leader. She is a member of the ISAC Council elected for the 2020–2024 term.
Webinar Summary
Visualization of multiparameter datasets is a staple task in the data analysis pipeline. Over last few years, multiple dimensionality reduction algorithms have been adopted for visual presentation of cytometry data to aid identification of novel cell populations and biological trends. In this webinar, we will discuss the basic principles of these approaches and identify benefits and drawbacks of several mainstream algorithms including popular variants of t-SNE and UMAP. We will specifically highlight the importance of hyperparameter optimization for cytometry datasets and practical considerations of choosing the suitable computational environment for your analysis.
Learning Objectives
- Familiarize the audience with recent advances in dimensionality reduction approaches in cytometry datasets.
- Introduce and discuss the basic principles of popular dimensionality reduction algorithms and compare their strengths and weaknesses.
- Demonstrate how computational analysis enhances the power of high parameter flow cytometry.
Who Should Attend
Cytometry practitioners and data analysts who encounter multidimensional datasets and employ algorithmic data analysis approaches or are interested in learning these methods
CMLE Credit: 1.0