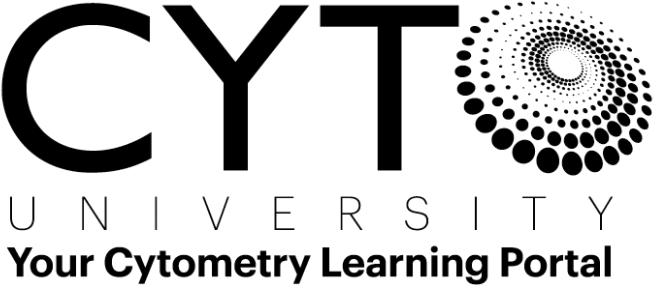
CYTO Virtual Interactive 2021 Oral Presentation - VIA for Generalized and Scalable Single-Cell Trajectory Inference Beyond Transcriptomic Data
-
Register
- Visitor - $50
- Bronze - Free!
- Silver - Free!
- Gold - Free!
- Platinum - Free!
- Bronze Lab Membership - Free!
- Silver Lab Membership - Free!
- Platinum Lab Membership - Free!
Overview
Inferring cellular trajectories is a critical task in single-cell data science and remains under-explored in the realm of cytometric data. However, accurate prediction of cell fates, and thereby biologically meaningful discovery using single-cell omic data, currently faces four challenges: (1) lack of robustness in accurate reconstruction of cell trajectories prevents detection of less populous lineages and renders hypothesis testing highly susceptible to user parameter choice; (2) lack of generalizability to infer disconnected, cyclic, or hybrid topologies without imposing restrictions on transitions and causality; (3) limited scalability on large data size not just in terms of runtime but in the preservation of global neighborhood relationships which are key to capturing transitional dynamics; and (4) restricted applicability of TI to a broader spectrum of single-cell data beyond transcriptomics, with existing methods predominantly designed for transcriptomic data.
We present VIA, a scalable trajectory inference algorithm that overcomes these limitations by using lazy-teleporting random walks combined with Markov chain Monte Carlo (MCMC) simulations to relax common constraints on graph traversal, and thereby accurately reconstruct complex cellular trajectories beyond tree-like pathways (e.g., cyclic or disconnected structures). Automated and robust lineage detection is facilitated by analysis of various node properties along a directed graph which capture the probabilistic transitions between cells. The properties of the lazy-teleporting walk preserve global neighborhood relationships when the data size scales and also improve the accuracy of inferred pseudotimes.
We first validate that VIA detects elusive lineages and less populous cell fates on various developmental data, including single-cell proteomic, epigenomic, multi-omic, and new in-house morphological datasets. VIA also efficiently unravels the fine-grained trajectories in the 1.3-million-cell atlas of mouse organogenesis without losing global connectivity at such high cell counts. This type of broad applicability of TI beyond transcriptomic analysis is increasingly critical, but existing methods have limitations contending with the disparity in the data structure (e.g., sparsity and dimensionality) across a variety of single-cell data types and are often designed with a view to only handling transcriptomic data.
We show that VIA is robust against the dimensionality drop (down to 10āsā100ās dimensions) in mass cytometry (proteomics) and imaging cytometry (morphological) data. For instance, VIA reconstructs the pseudotime underlying murine embryonic stem cells (ESCs) differentiating toward mesoderm cells in CyTOF data, where the lazy-teleporting MCMCs contribute to the high accuracy of inference. We hypothesize that VIA can also be applied to imaging cytometry to gain a mechanistic biophysical understanding of cellular progress. To this end, we profiled the biophysical and morphological phenotypes of single-cell live breast cancer cells with our recently developed high-throughput imaging flow cytometer, called FACED. Validated with in-situ fluorescence image capture, VIA reliably reconstructs the continuous cell-cycle progressions from G1-S-G2/M phase and reveals subtle changes in cell mass accumulation. VIA is available on GitHub at https://github.com/ShobiStasse... This link connects to tutorials highlighting compatibility with popular single-cell pipelines.
Speaker
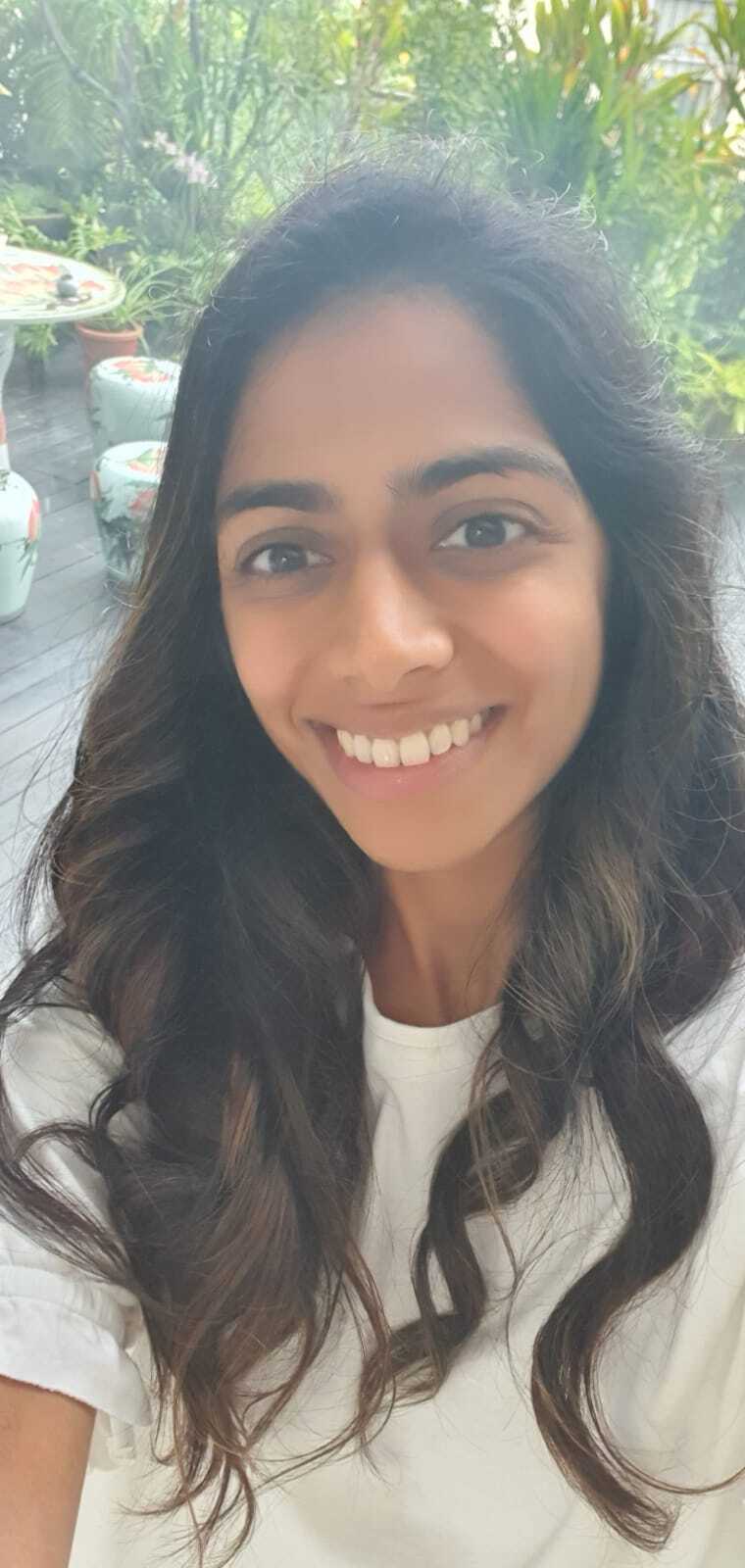
Shobana Stassen
Researcher
University of Hong Kong
Shobana Stassen received her BS with honors in engineering from Princeton University in 2010 and her Master of Science with distinction in electrical and electronic engineering from the University of Hong Kong in 2019. She is currently a researcher at the Applied Life Photonics group at the University of Hong Kong. Her research interests are primarily in the development graph based statistical methods suitable for multi-omic and scalable single-cell analysis.
CMLE Credit: 1.0