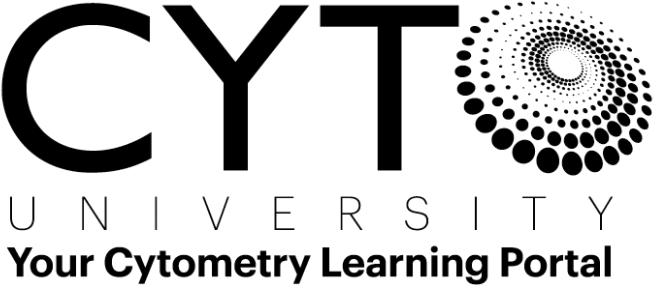
CYTO Virtual Interactive 2021 Oral Presentation - A Hitchhiker’s Guide to the Computational Analysis of Microbial Flow Cytometry Data
-
Register
- Visitor - $50
- Bronze - Free!
- Silver - Free!
- Gold - Free!
- Platinum - Free!
- Community Administrator - Free!
- ISAC Staff - Free!
- Bronze Lab Membership - Free!
- Silver Lab Membership - Free!
- Platinum Lab Membership - Free!
Overview
BACKGROUND: Flow cytometry is an important technology for the study of microbial communities. It is well-established in environmental and laboratory settings and is gaining traction in clinical settings as well. Microbial flow cytometry has a number of distinct characteristics that differ from those encountered in "typical" human polychromatic flow and mass cytometry data. As such, microbial flow cytometry data requires a dedicated set of bioinformatics tools that are a mix of general and microbial-specific computational algorithms. These are steadily becoming available. As such, microbiologists have the opportunity to benefit from a faster and more objective data analysis, with the ability to search for novel markers beyond the capability of manual gating.
RESULTS: The computational analysis of microbial flow cytometry data currently lacks a consensus set of guidelines. Here, we first summarize some the of the key properties of microbial flow cytometry and illustrate how the data differ from human polychromatic flow cytometry data. Next, we provide an overview of the full data analysis pipeline for microbial flow cytometry data, ranging from measurement to data interpretation. At each step, we highlight challenges and computational methods that are able to assist in the analysis. Extra attention is given to so-called cytometric fingerprinting approaches, which have the capacity to provide informative descriptions of microbial communities. Finally, we illustrate how this information can be used to characterize the status of microbial communities in an environmental and clinical context. To facilitate the uptake of computational methods for microbiology research, an example of a computational workflow in R can be found online at https://rprops.github.io/MSys_...
CONCLUSION: Microbiologists have the opportunity to benefit from the many computational methods that are being developed for the analysis of cytometry data. A number of challenges remain, such as the availability of shared microbial cytometry data sets, but also the exchange of information across cytometry disciplines. We hope with this overview to encourage the use of computational cytometry methods for microbiology research and connect different cytometry disciplines.
Speaker
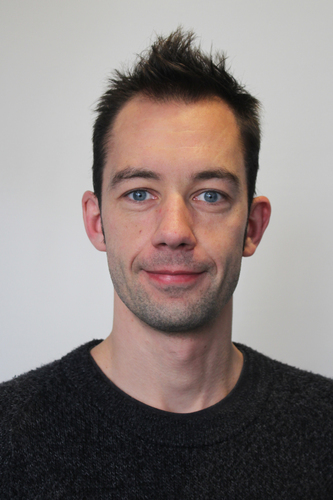
Peter Rubben
Postdoctoral Researcher
Flanders Marine Institute (VLIZ)
Peter Rubbens is a postdoctoral researcher at the Flanders Marine Institute (VLIZ) in Ostend, Belgium. He obtained his PhD at Ghent University in 2019. During his doctoral study, he investigated and specialized in data analysis and machine learning approaches for microbial flow cytometry data. Currently, his research has shifted to a quantitative study of microbial communities and their dynamics in marine environments using flow cytometry. In addition, he is interested in applications of machine learning for microbial ecology research.
CMLE Credit: 1.0